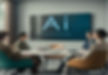
In the dynamic landscape of today's business world, harnessing the power of artificial intelligence (AI) has become a necessity for companies striving to stay ahead. It has become crucial for businesses to constantly innovate to unlock AI’s true potential. The introduction of Gen-AI and LLM’s (Large Language Models) has significantly increased productivity by eliminating redundant manual tasks. However publicly accessible AI tools can only go so deep with the limited data they can obtain. A groundbreaking idea on the horizon is the creation of custom LLM's (Generative Pre-trained Transformers) tailored specifically for individual companies. Trained on company data, this tool would provide more concise answers than publicly accessible LLM’s. This innovation holds the potential to transform the way organisations handle data, make decisions, and ultimately improve overall performance. In this blog, we shall go over the advantages of such a solution, its potential use cases, a preliminary strategy for development and the various implementation challenges.
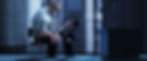
“A Company is like an enormous clock. It only works if all the cogs mesh together” as was said by Bob’s boss in The Incredibles. Hence to gauge the edge of a custom LLM would have would require us to assess its performance across the various domains of a company. Let’s look at a few.
1. Personalized Customer Interactions:
The relationship between a company and its clients can be significantly enhanced with a tailor-made LLM. By providing personalised responses to client inquiries and analysing client data to predict and meet customer needs, the LLM contributes to higher customer satisfaction. Efficient communication through LLM strengthens client relationships, fostering long-term partnerships.
2. Legal Compliance and Risk Mitigation:
Ensuring legal compliance is a paramount concern for any business. A customised LLM can play a pivotal role by reviewing legal documents and contracts to ensure they align with regulations. It can also answer legal queries related to contracts, compliance, and policies, providing real-time assistance and contributing to a more legally sound business environment.
3. Financial Management:
In the realm of financial management, a personalised LLM could revolutionise how companies handle their monetary affairs. By monitoring and analysing financial data in real-time, the LLM can provide instant reports and forecasts. Additionally, it could automate routine financial inquiries and transactions, freeing up valuable time for financial teams to focus on strategic decision-making.
4. Optimized Project Management:
In the dynamic landscape of project management, efficient communication is key. An LLM tailored for project management can facilitate updates and progress reports, assist in resource allocation, and streamline communication within project teams. This use case ensures that project-related information is easily accessible, leading to improved collaboration and successful project outcomes.
5. Employee Productivity and Engagement:
Employee engagement is crucial for a positive work environment. A chatbot based on the LLM designed for HR-related queries can foster this engagement by providing information on onboarding processes, training modules, and facilitating communication between employees and HR. Gathering employee feedback through the chatbot can also contribute to continuous improvement initiatives.
Moreover, A private LLM is customized to the company’s domain or use case, reducing dependency on major AI providers and ensuring cost efficiency. It also provides enhanced data privacy and security and can be aligned with open-source technologies if desired.
It becomes quite evident how the tailor-made LLM would boost a company’s efficiency. Now that we know “why” let’s look at “how” i.e. the rough steps to be taken to implement such a solution for a company:
Developing a personalised LLM involves a multi-faceted approach. First and foremost is the challenge of data integration. Establishing a secure method for aggregating and processing diverse data sets is crucial. This involves implementing data preprocessing techniques to ensure the relevance and accuracy of the integrated data.
The next step is to select the best model for the specific needs and fine-tune it to achieve the highest levels of accuracy and fluency. Examples of models include GPT-4, LLaMA, PaLM 2, Claude, DALL.E, Whisper, Stable Diffusion, phi - 2, Llama 2 etc. These models allow you to create your own LLM. However, Large language models may exhibit inconsistency, occasionally delivering accurate responses to queries, while at other times, they may simply reproduce arbitrary information gleaned from their training datasets. Hence, instead of training the model on the data itself, we can implement a RAG (Retrieval Augmented Generation) framework which incorporates external sources of knowledge, supplementing the internal information representation within the LLM. It guarantees that the model is equipped with up-to-date and dependable information, and users can access the sources used by the model, allowing them to verify the accuracy of its claims and build trust in its reliability.
User interface design is a critical aspect of customisation. Creating an intuitive and user-friendly interface ensures seamless interactions between users and the tools based on these LLM's. Implementing customisation options within the interface allows the solutions to align with the unique needs of each company, providing a tailored experience.
Say we understood how to make this LLM. However, implementing this in a large organization is quite a task and there are more aspects that must be taken care of before such a solution could be fully functional. Let us look at some obstacles that are essential to overcome:
Data Privacy Concerns: One of the foremost challenges in developing a customisable LLM is addressing data privacy concerns. Handling sensitive data, including financial, legal, and employee information, requires adherence to strict legal and ethical standards. For increased security, we can implement robust security protocols including granting companies full access control and the choice to store their data on their own servers. This proactive strategy substantially diminishes the risk of data breaches and unauthorized entry, ensuring the protection of confidential information.
Integration Complexity: Integrating the LLM with existing company systems can be complex. Overcoming challenges associated with integration requires close collaboration with IT teams. Developers must navigate compatibility issues and ensure a smooth implementation process to guarantee the LLM seamlessly integrates with various data sources and applications.
Quality Assurance: To ensure quality, we must adhere to a comprehensive development process that includes testing, validation, and continuous support and maintenance.
The potential benefits of implementing a customisable LLM tailored to a company's specific data points are vast. From improved efficiency to personalized interactions, businesses can unlock new levels of productivity and innovation. However, navigating challenges related to data privacy, quality assurance, and integration complexity is crucial for a successful implementation. As we step into the future of business, the integration of LLM's could very well be the key to unlocking unparalleled success and competitiveness in the corporate landscape.